🔹Fragmented Global AI Regulation: The USA, China, and the EU have adopted divergent AI regulatory models—sector-specific, state-controlled, and human-centric respectively—creating a complex global compliance landscape.
🔹USA’s Decentralized Approach: AI is governed through a mix of federal agency guidelines, executive orders, and state laws, prioritizing innovation and competitiveness but resulting in fragmented oversight.
🔹China’s Centralized Control: AI regulation is tightly state-managed with mandatory algorithm filings, ethical reviews, and content oversight, aiming to align innovation with national security and ideological goals.
🔹EU’s Unified Risk-Based Framework: The EU AI Act enforces a tiered risk classification system emphasizing human rights, ethics, and data privacy, but imposes high compliance burdens.
🔹Operational Implications: Companies face increased costs and complexity from navigating conflicting rules, requiring region-specific teams, documentation, and compliance processes.
🔹Regulatory Havens: Some countries (e.g., Argentina) can position themselves with minimal AI regulation to attract innovation and investment, offering reduced costs but potentially erecting barriers to global market access.
🔹Regulatory Sandboxes: Controlled testing environments offer a middle ground for innovation and oversight, helping startups and developing nations innovate responsibly.
🔹Strategic Imperatives for Firms: AI firms must balance innovation with compliance, tailor products for regional rules, monitor regulatory shifts, and integrate ethical safeguards early to remain competitive and compliant.
Artificial intelligence (AI) is revolutionizing how industries and societies function around the globe. Companies now face a delicate balancing act: drive rapid adoption and innovation while managing the emergent risks that come with such transformative technology. Governments around the world have yet to establish a consensus on the ‘regulatory approach’ while major jurisdictions—namely, the United States of America (USA), China, and the European Union (EU)—have adopted divergent regulatory models influenced by their political, economic, and cultural contexts. This reality is creating a complex compliance landscape for AI companies. To navigate fragmented regulations, AI firms must adapt to multiple legal regimes, driving up compliance costs and creating operational hurdles. In response, some turn to low-regulation jurisdictions (regulatory havens) or controlled testing environments (regulatory sandboxes), each offering distinct advantages and trade-offs. The future trajectory of AI firms will be shaped by their ability to navigate a fragmented and geopolitically charged regulatory landscape—balancing compliance across rival blocs while positioning for a possible convergence of global standards that could reshape both market access and strategic alliances.
Competing Rulebooks: AI Regulation in the USA, China, and the EU
United States of America
In the USA, the guiding principles for AI regulation are to promote technological innovation and economic competitiveness through collaborative policy development. Accordingly, the USA has adopted a decentralized approach, regulating AI through sector-specific rules involving federal agencies such as the National Institute of Standards and Technology (NIST), the Food and Drug Administration (FDA), and the Federal Trade Commission (FTC). For instance, the Securities and Exchange Commission (SEC) and the Defense Innovation Board of the US Department of Defense (DoD) have issued guidance for AI usage in their respective domains. Furthermore, a mix of federal executive actions and state legislation further governs AI development in the absence of a central AI regulatory body.
US AI regulatory landscape largely responds to the economic and strategic imperative of AI leadership, fostering a non-restrictive environment for AI firms.
While the overarching aim remains to stay ahead in the global AI arms race through technological advancement, the government has made efforts to ensure safety from AI systems. These include Executive Orders EO 13960 (2020)—Promoting the Use of Trustworthy Artificial Intelligence in the Federal Government, and EO 14110 (2023)—Safe, Secure, and Trustworthy Development and Use of Artificial Intelligence, alongside the non-legally binding Blueprint for an AI Bill of Rights (2022) and the Bipartisan House Task Force Report on Artificial Intelligence.
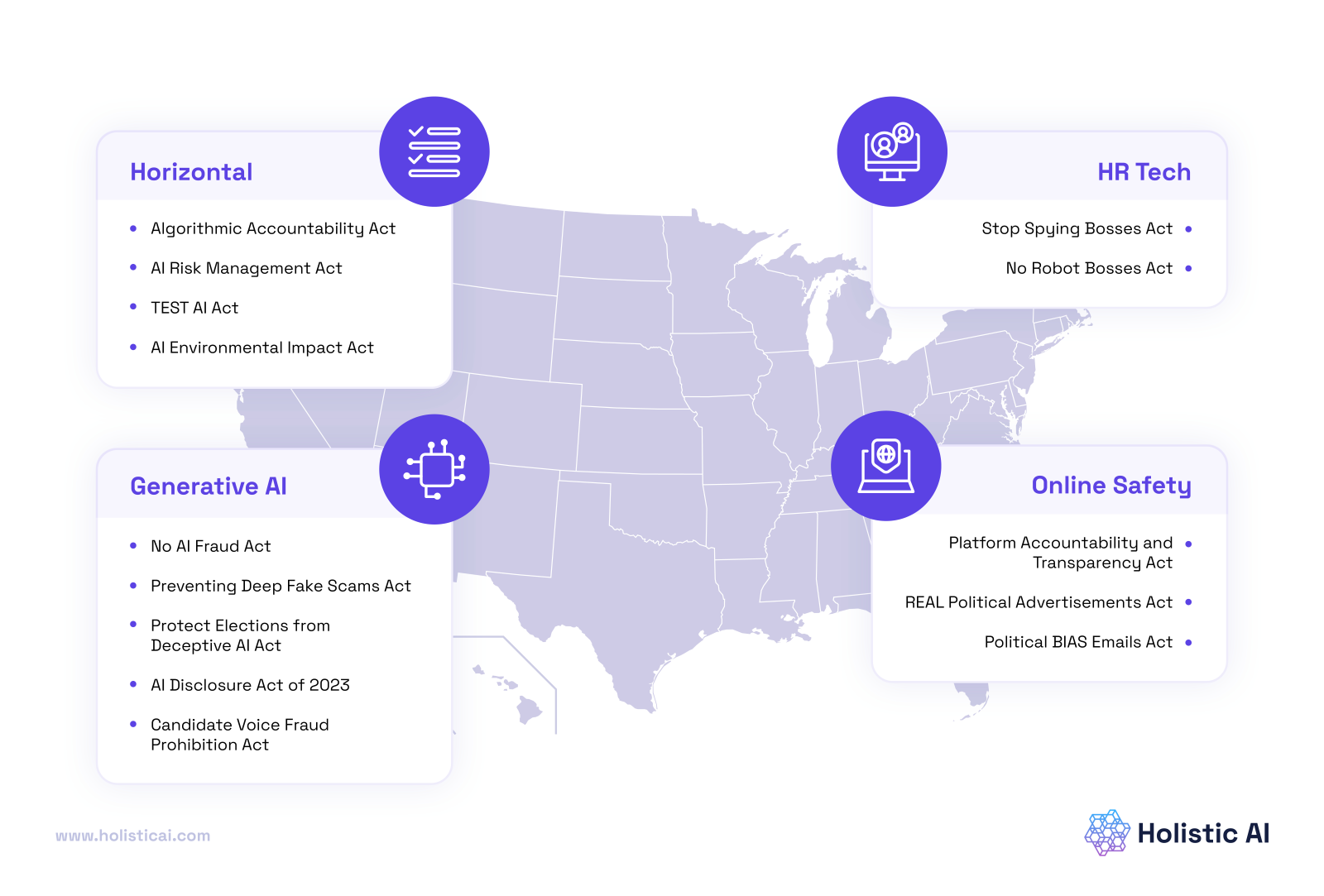
Hence, while regulations remain fragmented, government efforts address core themes across all frameworks, such as safety from AI systems, transparency, accountability, data privacy, protection from algorithmic bias, and an emphasis on human control over AI systems. In this sense, the US AI regulatory landscape largely responds to the economic and strategic imperative of AI leadership, fostering a non-restrictive environment for AI firms while remaining wary of potential risks and addressing them on an ad-hoc basis.
China
China’s approach is characterized by balancing innovation and economic competitiveness with strong state control. Here, the core regulatory aspects are shaped by state priorities, with the government recognizing AI as a national policy pillar. This has resulted in robust government oversight, mandatory algorithmic filing and reviews, labelling of AI content, misinformation control, security risk assessments, and AI education for workforce development.
Chinese regulations are determined by three main bureaucratic bodies with distinct but complementary roles. First, the Cyberspace Administration of China (CAC) focuses on public opinion and issues technology-specific regulations for algorithm recommendations, generative AI, and deep synthesis. Chinese authorities define deep synthesis as “technology utilizing generative and/or synthetic algorithms, such as deep learning and virtual reality, to produce text, graphics, audio, video, or virtual scenes.” Second, the China Academy of Information and Communications Technology (CAICT) develops tools for testing and certifying trustworthy AI systems. Finally, the Ministry of Science and Technology (MOST) establishes ethical principles for AI and creates technology ethics review boards within firms and research organizations.
The ultimate aim of the Chinese approach is to leverage institutional capabilities and foster innovation while maintaining state control over the development trajectory of AI.
Furthermore, several vital regulatory tools underpin China’s AI governance framework like the Interim Measures for Managing Generative AI Services, the Administrative Provisions on Deep Synthesis of Internet Information Services, the Trial Measures for the Ethical Review of Scientific and Technological Activities, and the Administrative Provisions on Algorithm Recommendations for Internet Information Services.
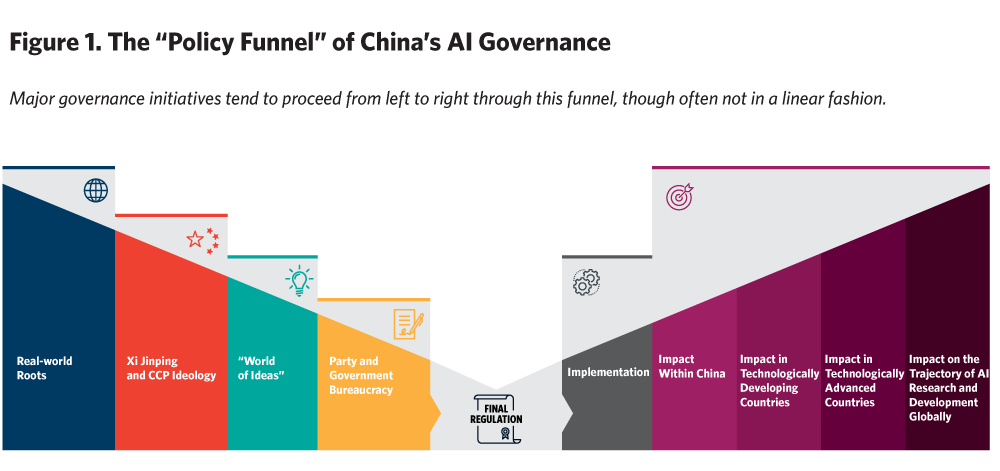
The ultimate aim of the Chinese approach is to leverage institutional capabilities and foster innovation while maintaining state control over the development trajectory of AI. Hence, while the AI ecosystem in China benefits from significant government support through investments in R&D and sector-specific applications, it is also tightly regulated in the context of national security, state control, and the government’s objectives to achieve technological leadership amid growing geopolitical competition with the USA.
European Union
The EU’s approach is characterized by a unified, human-centric development of AI. It aligns with human rights and aims for a positive societal impact, encompassing ethical, privacy, and non-discriminatory considerations. Simultaneously, it seeks to safeguard individuals against security risks. The EU enhances transparency and accountability through robust documentation, risk assessments, and human oversight. It also enforces safety standards that require testing and validation of AI systems—particularly in critical domains such as healthcare, logistics, and manufacturing. Furthermore, the EU prioritizes privacy and individual data protection by ensuring AI systems process personal data in compliance with the GDPR.
The EU enhances transparency and accountability through robust documentation, risk assessments, and human oversight.
In this respect, the EU AI Act enforces a horizontal legislative framework applicable across sectors and industries. Its comprehensive design aims for adaptability as technology advances, and provides a tiered risk approach: Unacceptable Risks—subject to outright prohibition; High Risks—subject to rigorous conformity assessments; Limited Risks—subject to transparency obligations; and Minimal Risks—subject to a voluntary code of conduct.
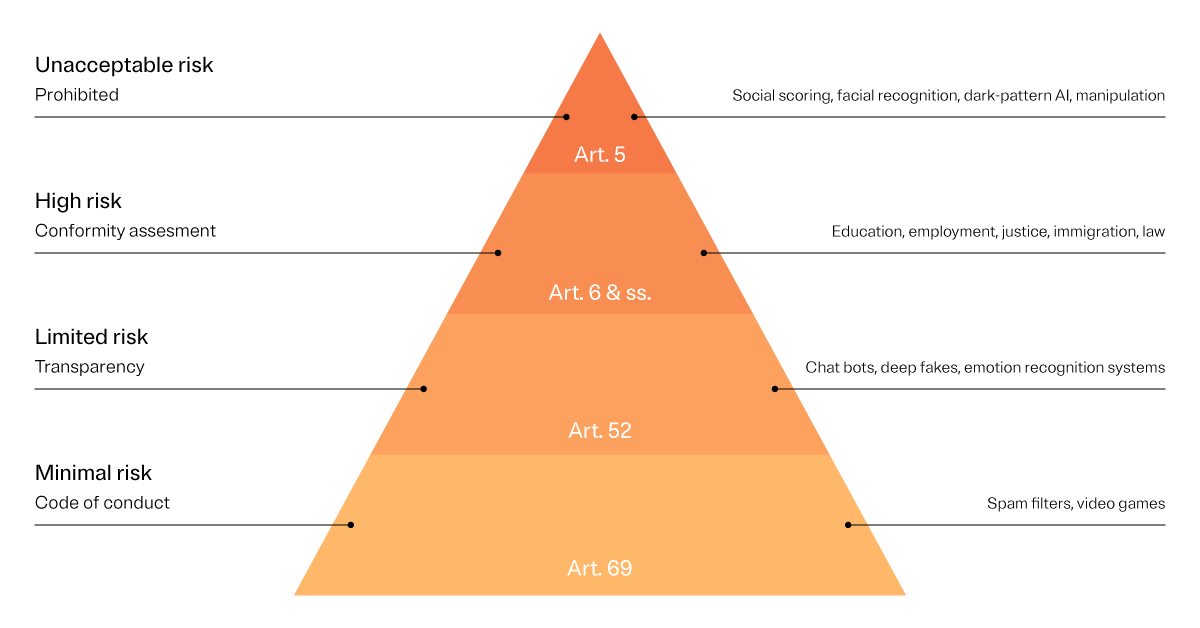
Operational Implications and Compliance Challenges
The USA’s patchwork and decentralized regulatory environment, with an emphasis on industry-wide self-regulation, creates a complex compliance challenge. While federal initiatives provide broad direction, state legislation focuses on local needs and concerns. For instance, California’s CCPA regulates the handling of personal data—including data used by AI systems—while Illinois' BIPA specifically protects individuals against the misuse of biometric data by AI-driven technologies. These differences create hurdles for AI firms as they navigate multiple, potentially overlapping regulatory frameworks across sectors and states. This dynamic has operational implications for firms, including increased compliance requirements—such as bias, privacy, and transparency audits—according to sector-specific rules. The result is higher operational costs and market limitations, as scaling nationwide becomes more difficult.
In China, the level of compliance requirements creates several challenges for AI companies. For example, comprehensive algorithm filing imposes a significant administrative burden, including determining which algorithms require filing, protecting commercial secrets, and meeting dual filing requirements if a company offers deep synthesis services and develops the underlying technology. Algorithm filing is a continuous obligation, as entities must update filings whenever there are amendments. Moreover, policy frameworks like the Generative AI Measures apply to services offered to the Chinese public, regardless of whether the provider is based in China, compelling international AI companies to comply even without a physical presence. Content oversight also creates obligations that may conflict with product designs or approaches permitted in other markets. While Chinese firms may be adept at maintaining conformity within strictly defined legal and regulatory parameters, foreign firms may struggle to maintain innovation and competitiveness in this jurisdiction.
The EU’s comprehensive approach to AI regulation puts it at the forefront of AI governance, potentially at the expense of innovation and competitiveness. The categorical risk framework requires tech firms to meet distinct compliance requirements. For example, if classified as an ‘unacceptable risk,’ certain AI applications are restricted in EU markets even if permitted elsewhere, while ‘high-risk’ applications require firms to invest substantial resources to meet EU-specific compliance standards. The designation of General Purpose AI systems—recognized for their broad application and associated risks—introduces an additional layer of compliance that firms must manage. The EU regulatory approach, built on the foundation of GDPR, has been criticized for being overly constraining for AI development and creating a competitive disadvantage for European AI startups compared to startups elsewhere.
‘High-risk’ applications require firms to invest substantial resources to meet EU-specific compliance standards.
Beyond direct operational implications, these divergent regulatory approaches create compliance burdens for companies operating in multiple jurisdictions. This dynamic compels multinational tech firms to maintain multiple dedicated compliance structures and protocols to fulfill the requirements of each regime. Furthermore, firms may face contradictory compliance requirements across jurisdictions. For example, China’s data localization mandate can put product design in direct conflict with data protection requirements in the EU, creating situations where a product design compliant in one regime compromises compliance in another.
Such dynamics require tech firms to establish region-specific compliance teams with specialized expertise in regulatory frameworks to manage different documentation and reporting processes. This increases operational complexity and can negatively affect organizational coherence. For instance, EU compliance requires extensive risk assessments, compliance in China demands coordination with the government for registration and security reviews, and compliance in the USA involves self-regulation across state legislation and sector-specific requirements.
Navigating these diverse and often conflicting regulatory landscapes imposes significant financial and operational costs on firms. The need to establish dedicated compliance teams, tailor product designs to region-specific rules, manage extensive documentation, and fulfill audit obligations diverts resources away from innovation and market expansion. Ultimately, these factors increase operational expenditures, limit economies of scale, and delay product launches or deployments. For startups and smaller firms in particular, such costs can become barriers to entry or expansion, inadvertently allowing only larger, well-resourced firms to dominate.
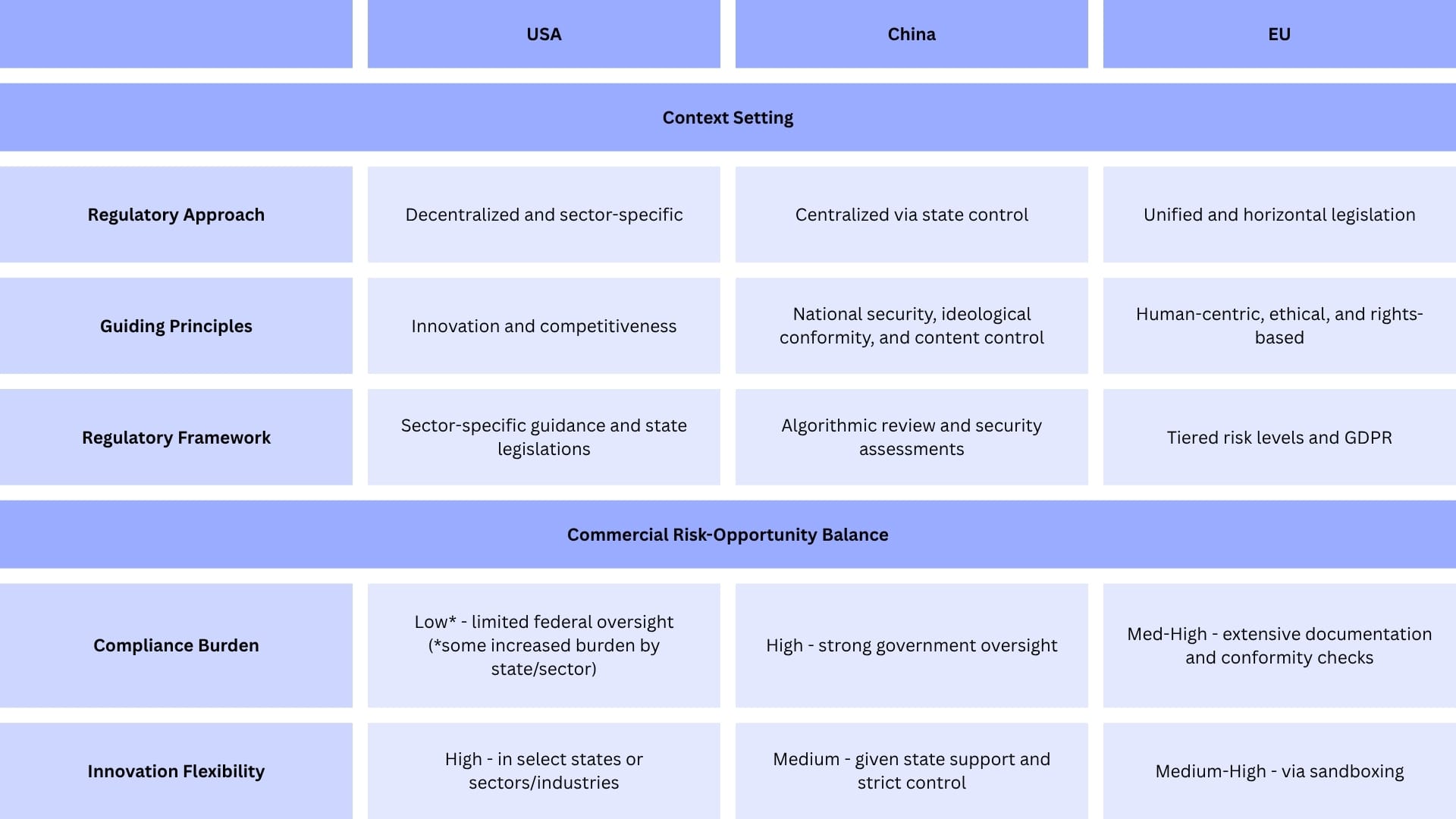
Innovation Dynamics: Regulatory Havens and Regulatory Sandboxes
Regulatory Havens
Regulatory havens are jurisdictions that offer relatively light regulations and less stringent safeguard standards regarding AI development. Countries that currently lack a comprehensive regulatory framework provide favorable operating environments and inadvertently foster a greater appetite for risk-taking.
Two main factors are driving this dynamic. First, strict regulatory frameworks indirectly make less regulated jurisdictions more attractive for AI development, as companies seek to maximize profits by taking advantage of permissive environments. Second, varying regulatory intensity allows some countries to strategically position themselves as alternatives to more restrictive jurisdictions, attracting investment and talent from companies seeking to avoid stringent environments. Argentina serves as an example of this strategy: President Javier Milei, by promoting low AI regulation, seeks to establish the country as “the world’s fourth AI hub.” His economic adviser Demian Reidel has stated that “extremely restrictive” rules have “killed AI” in Europe, and discussions in the US suggest a similar trend that might prompt companies to seek more favorable environments. Argentina’s concentrated efforts—through meetings and summits with industry leaders and investors—indicate a deliberate strategy to attract AI companies.
[Regulatory havens] are likely to attract increased investment from venture capital and private equity firms, given a business-friendly and relatively predictable environment.
As a result, companies may strategically relocate operations to jurisdictions that offer lower compliance costs, allowing them to maximize innovation. This creates competitive advantages for some regions but also raises concerns about regulatory arbitrage and the potential undermining of stricter regulations elsewhere, as well as other international initiatives toward AI governance.
For AI companies, regulatory havens offer several advantages. First, reduced compliance costs and administrative burdens due to light regulations can be particularly valuable for startups and smaller companies with limited resources. Second, these havens can accelerate time-to-market for AI products. Evidence from the fintech sector, where regulatory sandboxes have increased companies' average speed to market by 40% compared with the standard process, suggests that minimal AI regulations could yield similar benefits. Finally, these dynamics are likely to attract increased investment from venture capital and private equity firms, given a business-friendly and relatively predictable environment.
Beyond the benefits, AI companies also face risks when operating from a regulatory haven. First, AI products developed in such jurisdictions may encounter challenges when entering heavily regulated markets if they do not meet local standards. Second, lax regulations can attract competitors to the point of market saturation, eventually diminishing the original competitive advantage. Lastly, as countries’ economic priorities evolve, their status as regulatory havens can change. For instance, while the UK currently lacks regulation as stringent as the EU AI Act, the Information Commissioner’s Office has initiated enforcement actions against AI companies deploying products without proper risk assessments. In weighing these benefits and risks, companies must balance their reliance on regulatory flexibility against the potential drawbacks it might entail in other jurisdictions.
Regulatory Sandboxes
Regulatory sandboxes offer a viable alternative to operating from regulatory havens. Sandboxes are controlled environments that allow the development and testing of new AI technologies with reduced regulatory constraints under a regulator’s supervision. Serving as a pragmatic middle path between rigid regulatory restrictions and unrestricted innovation, they enable developers to explore experimental avenues and gain critical insights into new AI capabilities. Such collaborative arrangements accelerate responsible AI development while ensuring adequate safeguards, regulatory observation, and evaluation in near-real-world scenarios without being immediately subject to full regulatory enforcement.
Importantly, regulatory sandboxes address a fundamental challenge with all current attempts to effectively regulate AI: legislation rarely keeps pace with rapid developments in AI. This creates regulatory gaps and can leave emerging risks unaddressed. Sandboxes, however, offer a more collaborative approach; the model places regulators in a feedback loop alongside AI development. From an innovation perspective, it moves beyond the traditional binary of free-market-driven innovation versus government regulation, allowing the integration of consumer protection safeguards during development. This mechanism is especially effective for developing or underdeveloped countries with limited regulatory capacity and exposure to AI, bridging the gap between policy requirements and practical regulation.
Sandboxes, however, offer a more collaborative approach; the model places regulators in a feedback loop alongside AI development.
For AI developers, sandboxes offer multiple benefits, including reduced time-to-market due to earlier regulatory feedback and greater legal certainty. This enables companies to identify and preemptively address compliance issues, potentially avoiding costly redesigns or market withdrawals. The structure is particularly beneficial to resource-limited enterprises and startups, creating a more level playing field by reducing overall compliance costs. Moreover, early identification of compliance issues in a sandbox scenario is especially helpful for companies developing high-risk AI systems that require extensive documentation, conformity evaluations, and risk assessments. Furthermore, companies may enhance their reputation as responsible AI innovators committed to ethical development, influence regulations by providing feedback to regulatory interpretations and standards, and enjoy potential approval advantages when regulations formally take effect.
From Complexity to Action: Seven Priorities for AI Firms
Until global convergence of AI regulation becomes a reality, AI firms must operate within a fragmented and divergent landscape. Successfully navigating these complexities will demand strategic foresight, careful balancing of regulatory flexibility against market access, and thoughtful management of reputational and long-term stability risks. Accordingly, we can identify seven immediate priorities facing AI firms.
Primarily, operational strategies should strike a balance between minimizing compliance costs and sustaining innovation, ensuring that regulatory obligations do not hinder the overall progress of firms. This can be achieved by embedding compliance functions within the product development process and understanding the legal perspectives of the AI system being developed.
Firms then must prioritize key markets that suit their models, and where the compliance cost and burden are justified by commercial value. This can be ensured by conducting a cost-benefit analysis of entering and/or expanding in particular jurisdictions. Additionally, firms may consider postponing entry into certain markets until the associated compliance requirements have eased. Furthermore, splitting AI products and services into multiple versions tailored to specific regulatory regimes can facilitate market access. This would involve regulation-specific features as well as maintaining jurisdiction-specific datasets and user controls.
To stay ahead in the AI race, firms must systematically track regulatory shifts, legislative developments, enforcement actions, and emerging standards to ensure timely, strategic decision making. Firms can achieve this by establishing dedicated internal teams or partnering with external experts to manage ongoing regulatory watch functions. Insights from these watch functions should directly inform decision-making, product development, and compliance efforts, enabling firms to act preemptively when regulatory shifts are detected early. Further, regularly engaging or partnering with specialized advisory firms delivers the crucial insights needed to navigate uncertainties such as legal liabilities, reputational risks, and market dynamics. Leveraging their expertise, these firms can pinpoint legal gray areas, interpret ambiguous regulations, develop best practices, deliver comprehensive risk assessments, and advise on compliance strategies and resource allocation.
Furthermore, firms should allocate sufficient compliance resources when developing high-risk AI systems to mitigate legal liabilities and prevent operational disruptions. Examples of such systems, including biometrics, employee-hiring algorithms, and autonomous platforms, demand a risk-based compliance framework that includes comprehensive documentation, impact assessments, manual oversight, and post-deployment monitoring. Internal structures such as risk mitigation teams, review committees, or AI ethics boards are instrumental in ensuring alignment with both regulatory obligations and ethical standards.
Finally, by integrating ethical and regulatory requirements from the outset of system design, firms can position themselves for compliance while creating more trustworthy AI solutions. Embedding compliance checkpoints, from data collection and model training to deployment, helps avoid costly redesigns and mitigate reputational risks. This approach demands a multidisciplinary team of legal, ethical, and domain experts who conduct risk assessments, bias testing, and security audits throughout the development process. Aligning frontend and backend design with evolving standards of transparency, accountability, and user rights not only supports compliance but also strengthens stakeholder trust.